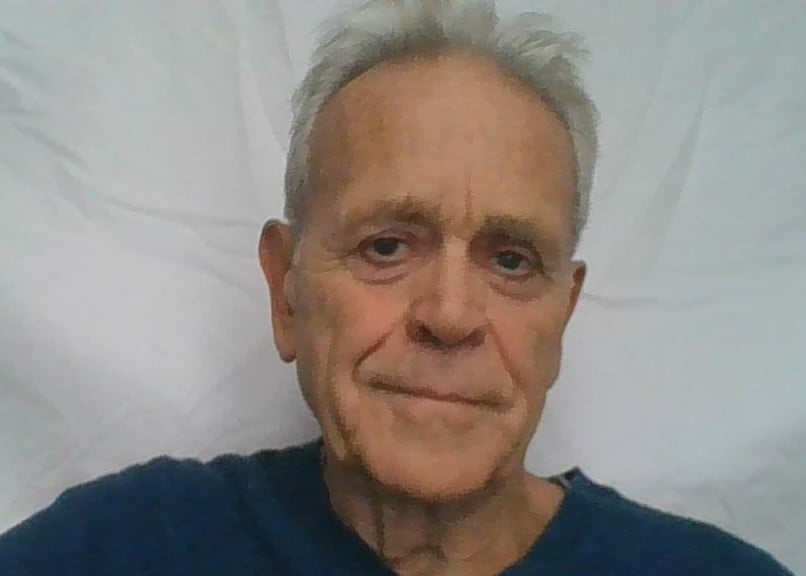
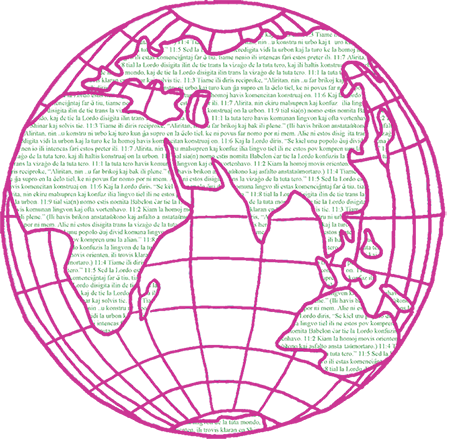
Translation automation has recently experienced a major shakeup – the emergence of neural MT*. This marks the start of a new journey of exploration into the opportunities and limitations of machine learning (ML) in translation and language technology more generally.
Two features stand out in this spectacular début. One is the rapid creation of a new ecosystem. The other is a pressing need to disrupt the current approach to sourcing language data for translation automation. The enriched ecosystem can help build a better language data pipeline for the entire industry, as both will depend on improved technology solutions.
A New MT Ecosystem
Experimentation in neural MT has brought together teams from research, development, media, and production in a far more fluid and visible alliance of knowledge-sharing, testing, feedback, and implementation than was previously possible in the MT space.
Statistical MT began to make an impact on practical translation tasks about ten years ago and developed independently from the media and most other technology developments. Neural, on the other hand, reached alert techie eyeballs in 2014 due to a hardware breakthrough, became the focus of a community of artificial intelligence (AI) research in 2015, and launched as an industry solution in 2016, attracting pure play MT companies and big Internet players alike. The pace of change has been phenomenal.
Today, the existence of this ecosystem means there is regular media coverage of the field, multiple events where the technology is demoed, discussed, doubted about and democratized, and a testing campaign plus take-up by MT suppliers, LSPs and others all over the world.
It also means (as we shall see later) that more testing and evaluation can operate on “richer” language data sources, ideally from industry and other real-world sources so that research can build engines directly that are tested on a broader range of data than that used today.
And this new buzz around MT could also lead to a further benefit: a richer dialogue between MT and other fields of computation and AI. From knowledge processing to quantum hardware, this could open exciting new vistas for translation automation beyond the usual concern with quality, time and cost alone: think of spoken translation opportunities, text to pictures/conversations, translation/summarization, translation as augmented reality support, languages as games, the challenges of the Human Language Project, and much more.
Language as Data
The second feature of this renewed momentum in MT is the conceptual shift from Big Data to language data – the stuff these famous ML algorithms work on to do their black magic. There is naturally much debate about how much data a given neural system needs to build a baseline engine that can drive a translation production line for a given semantic domain. Responses depend on multiple parameters and issues: a lot of data for a baseline, much less than before for a domain-specific? Experimentation is all. But both quality and quantity are key parameters: the real question is, how can the availability of language data in general be optimized to enable better translation?
Big Data is a key by-product of four decades or so of intensive digitization of massive amounts of spoken and written information. Language data, however, as a valued asset for translation automation, has never been “big” in the Big Data sense. Their value does not come from expanding continuous series such as time points, or sets of increasing/decreasing numerical values, or even from tokens of individual words expressing sentiment or bias. It comes exclusively from (fairly stable) syntactic-semantic patterns of words found in parallel texts.
Relatively few patterns (at least from a Big Data perspective) have the power to position many different words in much-repeated sets of phrases and sentences. The fundamental challenge for MT, therefore, has been to obtain lots of parallel versions of the same text to train a new engine, not lots of “new” series of data. Now that we have an ecosystem of players who are leveraging ML software for translation applications, the time is ripe to look more closely at organizing the language data space more efficiently to benefit more from these new neural discoveries.
Google Director of Research Peter Norvig said recently in a video about the future of AI/ML in general that although there is a growing range of tools for building software (e.g. the neural networks), “we have no tools for dealing with data." That is: tools to build data, and correct, verify, and check them for bias, as their use in AI expands. In the case of translation, the rapid creation of an MT ecosystem is creating a new need to develop tools for “dealing with language data” – improving data quality and scope automatically, by learning through the ecosystem. And transforming language data from today’s sourcing problem (“where can I find the sort of language data I need to train my engine?”) into a more automated supply line.
Silos to Marketplaces
It is common to refer to data as the “oil” of the digital age. But as The Economist said in an excellent recent briefing, unlike oil, data is not yet a marketplace and in many ways does not function like the energy economy. “The data economy…will consist of thriving markets for bits and bytes. But as it stands, it is mostly a collection of independent silos.” An excellent characterization of the state of language data today.
For example, LSPs tend to use data from their customers for their customers, and do not share it outside of that relationship, for example in exchange for similar data from a competitor or collaborator. Data ownership and copyright issues largely rule over this obsession with ring-fencing language data from its potential users.
At the same time, there are a number of language data repositories available (either free or paying) ranging from Linguistic Data Consortium (LDC) and Open Language Archives Community (OLAC) via DGT-Translation Memory and Opus to MyMemory, Tatoeba and the TAUS Data Cloud, sometimes offering versions of the same data sets. The Web itself also offers a (risky) source of parallel language data open to any crawler. Yet very few of these siloes share any common principles of quality evaluation or internal standardization. And if there are any effective tools for Norvig’s “building, correcting, and verifying” of data, they are not typically shared by the community.
Yet it should be possible to build a marketplace to trade the abundance of language data that exist in these siloes, and in its simplest formulation, enable users (from researchers to MT suppliers) to exchange (or buy and sell) high-quality language data in a far more efficient way than is often the case today.
What’s Special about Language Data
One complicating factor is that data, unlike oil, is not a commodity: As The Economist points out, data is usually not fungible because data streams cannot simply be exchanged. Each, therefore, has its own specific value. But in the case of parallel language data, the uniqueness of a data string is its semantic relevance – its capacity to mean the same as another string of data in another language. Structured data sets of numerical information are clearly not pure commodities (because of each unit matters), but natural language is redundant to a certain degree, and this renders it more commodity-like as data than a time series of financial data or an automobile’s travel log. The more commodity-like a resource, the more easily it can be exchanged in a marketplace, as it is easier to put a price on it.
The other key complication in setting up a language data market is the nature of data ownership. In most segments of the digital industry, it is much easier for one company to simply buy out another for their data, than to trade with that company in a marketplace. However, it should be possible reach an agreement on the market value of language data sets by applying technology solutions such as anonymization and info-neutralizing that “transform” the data from semantically sensitive information into semantically neutral content. It can then be usable in MT operations as patterns of words, not as references to real-world entities.
In addition, this marketplace will have to operate according to shared standards in terms of the data exchanged. These would go beyond the current metadata/file format level of description and provide a thorough digital footprint of the data set, capable of informing purchasers about multiple technical features of the contents relevant to the type of ML engine or algorithms that will operate on it.
This, in turn, would create a need for language data “factories” able to prepare a computationally more sophisticated product for potential bidders. The emergence of these services around the translation process will first appear to raise the cost of translation. But ultimately this should pay off by enabling the massive expansion in the number of suppliers able to build engines and benefit from high-quality, tradeable language data in a “flatter” business configuration.
7 minute read