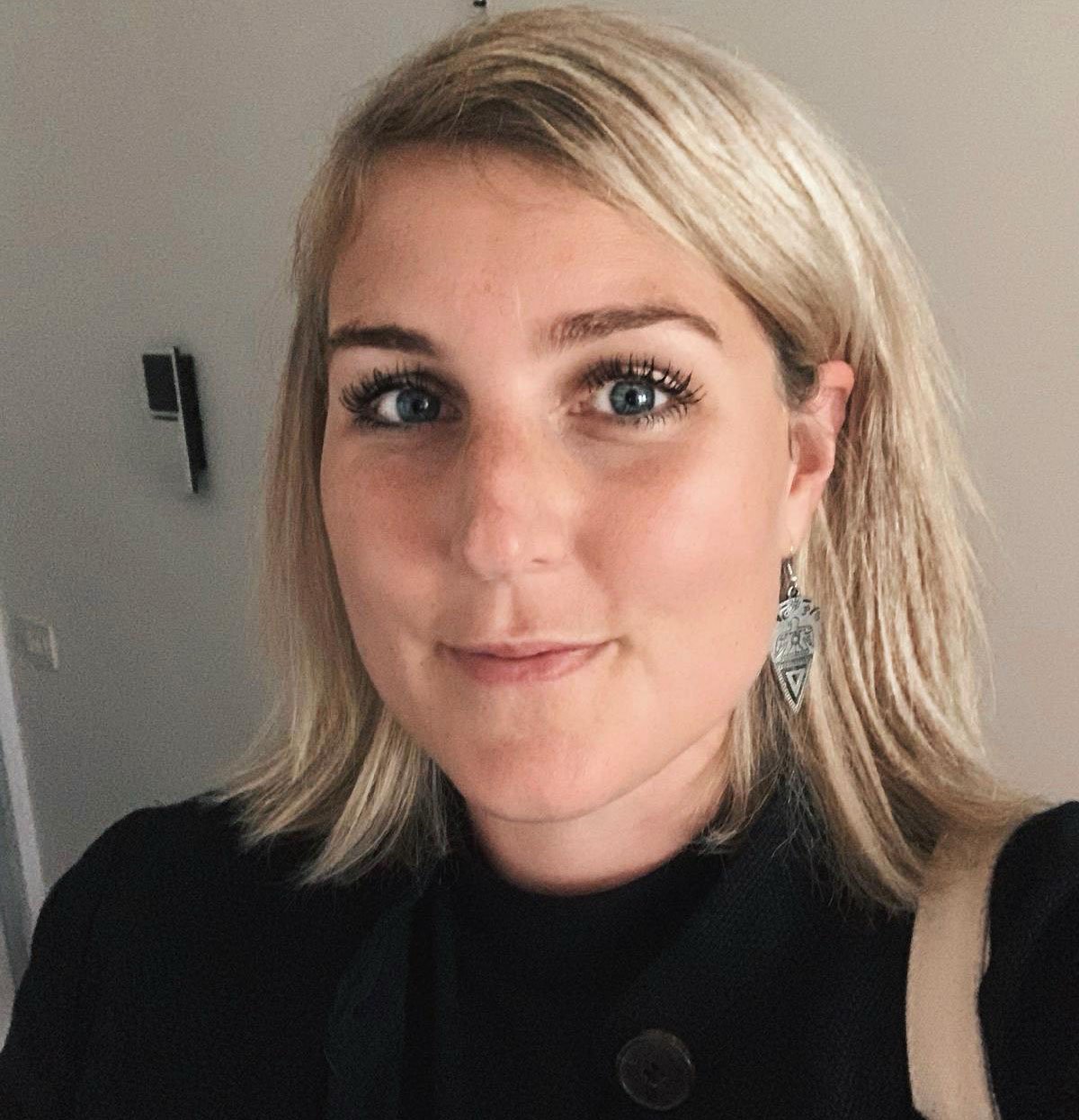
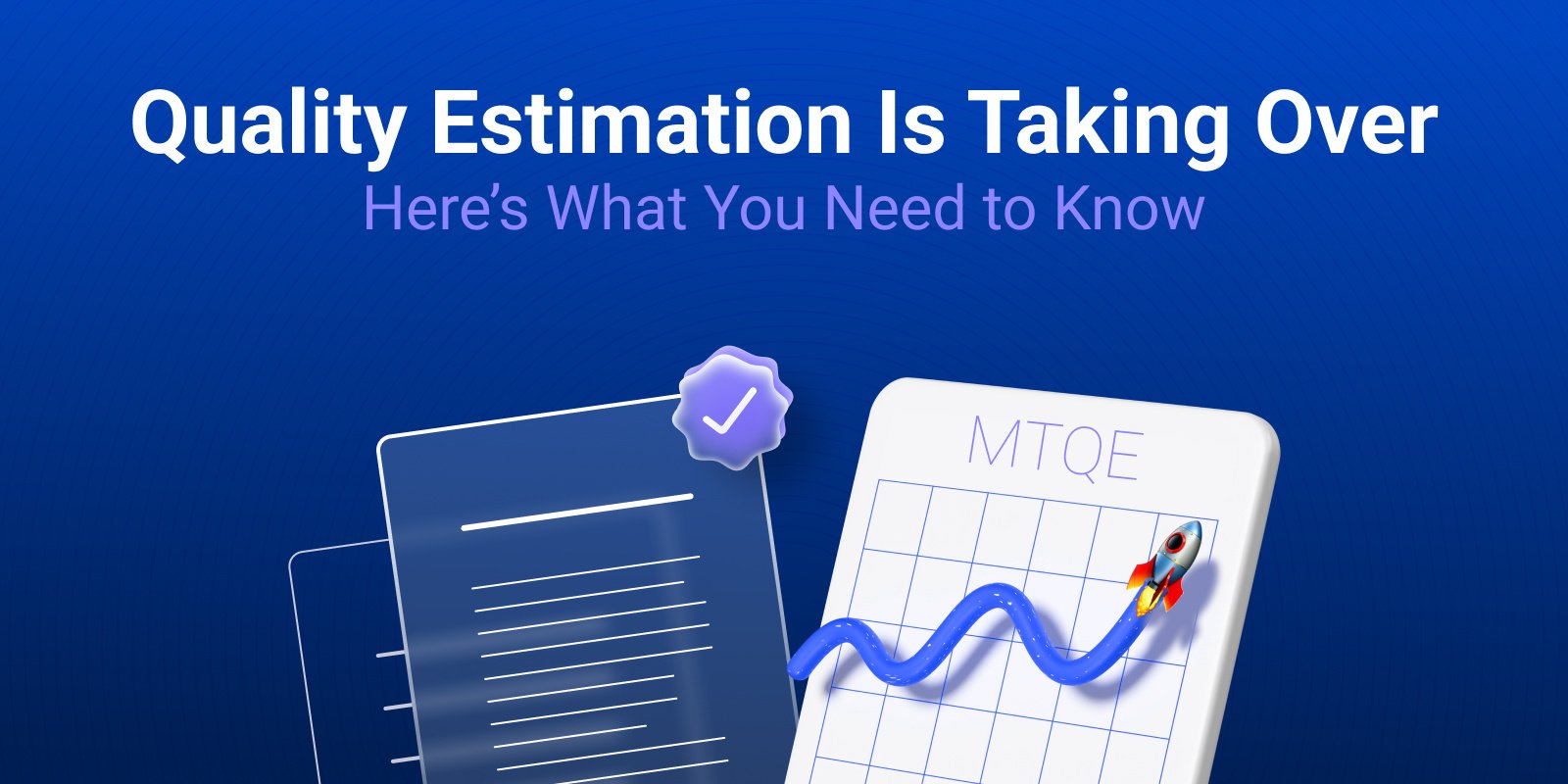
Earlier this year, our CEO Jaap van der Meer predicted that “2025 will be the year of MT Quality Estimation”, explaining why this is an essential technology that helps you take full control over your automated AI-driven translation process. A month into the year, we’re already seeing that prediction take shape.
With MT deeply embedded in translation processes, using post-editing already gained cost savings of up to 50%. But as Jaap pointed out, the next step is leveraging quality estimation to push those savings even further.
QE Taking Over the Market
The rise of Quality Estimation is being recognized across the industry. CSA Research has published a technology briefing about the TAUS EPIC Solutions, highlighting the growing role of QE in general in translation workflows. This shift is reflected also in the increasing number of LSPs and enterprises actively testing and implementing QE solutions.
At TAUS, we’re seeing more users every week that are looking to learn more about QE and start to experiment with our free trial and demo interface. We see a high interest from LSPs as well as enterprises wanting to fine-tune their workflows, and unlock new efficiency gains. As awareness grows and real-world use cases continue to prove its value, QE is quickly becoming a must-have tool for organizations looking to scale their translation operations.
Since QE is quite a novel technology, it’s not strange that many users are still a bit unclear about how it all works. Specifically for that, TAUS has provided an online QE Guide that discusses all the basics. The most challenging bit for many users is understanding the QE scores. What kind of errors are recognized and penalized? What actions do I take based on the quality scores? This Real-World Guide to QE Scoring shares many examples and best practices for businesses to kick off their QE endeavors in an informed way.
Seeing QE in Action
Automated QE, like what we offer through the EPIC API, enables real-time scoring and error detection for MT output. This means catching polysemy errors, omissions, number mismatches, spelling mistakes, tone inconsistencies, and more… before human reviewers even step in. The result? A more efficient, scalable, and cost-effective workflow that reduces post-editing efforts by up to 80%.
And it’s not just a promise. Companies like Uber, Blend, and Milengo are already benefiting from QE to streamline their translation processes, with cost savings of up to 80% reported. You can read more about their experiences and results in our case studies here.
Additionally, Kobalt Languages has recently shared a blog on how QE changes the game for them when it comes to post-editing workflows.
The Bigger Picture: AI-Driven Orchestration
Chris Wendt added another perspective in a comment on Jaap’s LinkedIn article: the future of translation involves orchestration. Smaller, dedicated language models (SLMs) will handle translation, while larger AI models will fact-check, ensure consistency, and refine domain accuracy. Quality estimation will be at the core of this new ecosystem, providing the crucial layer of automation needed for an AI-powered translation pipeline that’s both efficient and reliable.
Quality Estimation is the New MT
Just as MT disrupted the traditional translation workflows, QE is reshaping and automating post-MT production. It’s scalable, adaptable, and gives you full control over your translation quality.